The emerging Internet of Things (IoT) offers opportunities to improve how we design, measure and operate buildings. A research team at UC Berkeley’s Center for the Built Environment (CBE) conducted a six-month field demonstration of a system using IoT-connected office chairs, with integrated heating and cooling, that yielded valuable innovations for both building occupants and the research community. Results demonstrated high levels of comfort seen in few buildings. In addition, the data from occupants’ use of the chairs can be used to predict thermal comfort more accurately than methods previously available.
Understanding human comfort using empirical modeling methods
Thermal comfort affects people’s satisfaction, health, and productivity; yet in practice, most workplaces fall short in terms of providing conditions people find to be comfortable. To understand what makes a space comfortable, researchers have developed empirical models to represent our perception of thermal comfort in terms of personal and environmental factors. There are two comfort models that underpin the current practice of comfort management in buildings: the predicted mean vote (PMV) and the ‘adaptive’ model that is relevant for naturally ventilated buildings. Despite their wide adoption into international standards, these models have inherent limitations. For example, they are not accurate when applied to a small group of people, because they are designed to predict the average comfort of a population. Also, practical application of the PMV model in occupied buildings is not feasible, as it requires many specific inputs — temperature, air speed, metabolic rate, clothing insulation — that are costly and difficult to obtain in real-world settings.
Personal comfort models: A new paradigm for occupant-centric control
To overcome these drawbacks, CBE’s research team has proposed a new modeling approach called personal comfort models. A personal comfort model predicts people’s thermal comfort responses, specific to each individual, instead of the average response of a large population. This innovation resulted from a field study in which office workers were able to heat or cool themselves, using ‘personal comfort’ office chairs, that had been specially outfitted by the research team with user-friendly, battery powered, heating and cooling elements. These IoT enabled chairs allowed the research team to collect large amounts of data about the chair usage, space temperature, and other factors. Analysis conducted by Joyce Kim as part of her recently completed PhD dissertation led to an entirely new approach to comfort modeling, combining the IoT data with machine learning to generate individual-specific and context-relevant comfort predictions. In the future, the new personal comfort models may be used to provide individualized comfort feedback for the control of mechanical systems, leading to improved occupant satisfaction and energy efficiency, an approach that can be used in both buildings and vehicles. The research team believes this can fundamentally improve on today’s one-size-fits-all approach to space conditioning.
Published results and outlook
The CBE research team has published two journal papers on the topic of personal comfort models. The first paper proposes the new modeling framework, based on decades of thermal comfort and machine learning research. The paper includes a thorough review of existing literature on personal comfort models, methodologies to incorporate IoT and machine learning, and system architecture for indoor environmental controls. This is followed by a discussion on how such models can be integrated into building design, control, and standards. Read the journal paper or open access version via eScholarship for more information.
The second paper demonstrates how the new framework can be implemented, testing a set of personal comfort models based on CBE’s field study data collected from 38 office workers. Analysis was conducted using several non-traditional thermal comfort variables observed during the field test — thermal control ‘behavior,’ time factors, and system control settings — in combination with six machine learning algorithms. The personal comfort models were determined to be more accurate than conventional comfort models, with a median accuracy of 73%, compared to 51% for conventional models. They also showed that individuals’ thermal control behavior — chair heating or cooling settings — was a strong predictor of individuals’ thermal preference, producing the best predictive accuracy across all other variable types used for the model development (e.g., indoor or outdoor environment, mechanical system settings, date and time). Detailed results are in the published journal paper or an open access via eScholarship.
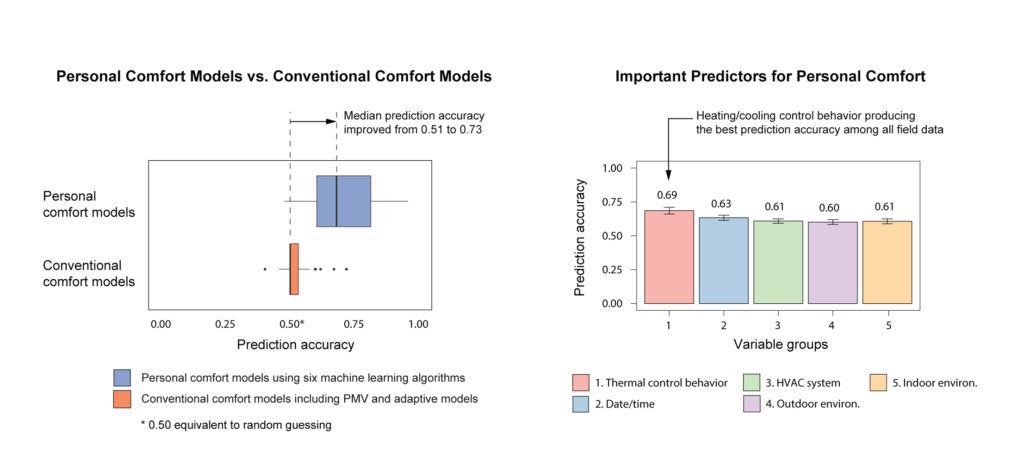
Predictive accuracy of personal comfort models developed for 38 office workers is shown above. The box plots on the left compare accuracy of personal comfort models and conventional comfort models. The box plots on the right compare accuracy based on variable groups.
The findings of this research may be used to better reflect individuals’ thermal requirements in everyday comfort management, for example, by informing the control of HVAC systems with more representative, data-driven temperature settings. In most commercial buildings there are limits to how much centralized systems can respond to individuals’ feedback, however these may be overcome with individualized control of thermostats, or emerging personal control systems (PCS) such as the CBE chair prototypes used in the study, providing individual heating and cooling. In the future, IoT-enabled buildings and such devices may interact with other systems to provide coordinated comfort solutions that can lead to greater satisfaction and efficiency.
References and acknowledgements
J. Kim, S. Schiavon, G. Brager (2018). Personal comfort models – A new paradigm in thermal comfort. Building and Environment. Publisher version or open access via eScholarship
J. Kim, Y. Zhou, S. Schiavon, P. Raftery, G. Brager (2018). Personal comfort models: Predicting individuals’ thermal preference using occupant heating and cooling behavior and machine learning. Building and Environment. Publisher version or open access via eScholarship
This research was funded by the Center for the Built Environment at UC Berkeley, the California Energy Commission Public Interest Energy Research (PIER) Buildings Program under Contract PIR-12-026, and the Republic of Singapore’s National Research Foundation through a grant to the Berkeley Education Alliance for Research in Singapore (BEARS) for the Singapore-Berkeley Building Efficiency and Sustainability in the Tropics (SinBerBEST) Program.